Unpopular Science - 241020 "What" vs "How"
AI is just one of many changes that is forcing us to confront deeper questions
As I integrate LLMs more into my daily life, I’m finding too many basic assumptions that need to be revisited.
This is a first draft that I’m sending to my trusted subscribers first. I’d appreciate any comments, (especially critical ones!)
Something big is happening
Even people who notice the dramatic scope of changes find it hard to articulate the level of uncertainty any of us has about the future. Here are a few of my thoughts about the biggest factors:
“Disintermediation”
I don’t like the term “partisanship”, which is over-used, but it’s clear that the US (and other western democracies) are dividing into mutually-combative camps. It’s traditional to say “left vs right” or “liberal vs conservative” but it’s clear that a simple label doesn’t capture the many contradictions. In US politics, the Democrat nominee is endorsed by a slate of military officials from previous Republican administrations, while the Republican is endorsed by people who, until recently were well-known and proudly Democratic Party officials.
A better label is something like “insiders vs outsiders” or “elites vs non-elites”. Martin Gurri’s excellent book Revolt of the Public blames the internet for amplifying outsider voices in ways that the insiders can’t control. Insiders, being human, have never been perfect, so when it’s too easy to expose their faults it leads to a plunge in trust.
Meanwhile, the “mainstream” media sources, forced out of their advertising-based business models, have to align with a “cause” in order to attract subscribers. Successful news sources find a niche among like-minded people willing to support the overall mission, even if it’s at the expense of neutrality. Why pay money to the New York Times if you disagree with them?
In other words, somehow the insiders, including media, are being disintermediated. What used to be a centralized, society-wide collection of acceptable beliefs is now being decentralized. The New York Times doesn’t agree with Joe Rogan because neither of them accepts the other as a mediator of which parts of the world deserve attention.
Attention is waning
Many people have noticed the apparent change in attention spans: Everyone’s on their screens. 40/% of young adults get their news from TikTok . Some of can remember when finding a basic fact required a trip to an almanac or encyclopedia. Few of read maps anymore; we literally don’t care where we are or where we’re going.
The new AI features on your phone will not help. Home cooks no longer need to read cookbooks—why bother, when ChatGPT can assemble a recipe based on your currently-available ingredients.
Along with this comes the deluge of fabricated images and audio, most of it extremely believable and lifelike. The end result is we’re learning not to trust what we see or hear as much as who it comes from. As Arnold Kling says “we decide what to believe by deciding who to believe.”
AI and the increased advantage of ‘what’ over ‘how’
I’ve been calling this “what” vs “how”, for lack of a better way to differentiate between the purpose behind an action and the action itself.
Until the arrival of generative AI, you had an advantage over other people if you had better “how” skills—you knew “how” to write a compelling Powerpoint or a spreadsheet or a C program, or maybe you knew “how” to translate from Japanese to English. These are skills that you can learn and apply on behalf of other people—the people who know “what” to do.
Your boss knows “what” to do, and he needs people who know “how” to do it. He’ll hire the best people he can find at doing the “how”. Right now, you happen to be one that he picked.
But now AI can do the “how”, often better than anyone. Who cares if you’re the best C programmer or Japanese translator? Now what matters is somebody who knows what to program or what to translate.
A doctor whose only skill is knowing “how” to cure a patient’s medical problem is rapidly becoming unnecessary. There are important soft skills of course (e.g. empathy) but that can be done by a nurse or assistant using the AI. In fact, it’s already possible for the patient himself to do the “how” (diagnosis).
If you had an army of AI bots that know “how” to report the news, then “what” you could do is maybe start your own newspaper.
If you knew “how” to write any app or game you want, then the problem is “what” game to write.
So how do you become better at the “what”?
I’m doing my own deep dive into personal science in an attempt to answer that question. ChatGPT knows “how” to do just about any self-tracking analysis I want. So “what” do I want?
Ten Rules for Personal Science
Bear with me as I struggle to put the overall concepts more concisely, but here’s my first draft:
Freedom of speech. More precisely, we need freedom to read and listen to anyone.1
Make up your own mind. Even if you rely on an expert, you still have to choose whichexpert.
Experts are often wrong. It’s important to listen to anyone who has more experience than you do, but don’t rely on somebody else for the final word.
You’re not an average. You’re you. “Treat the patient in front of you, not the average”.
Give extra weight to your own personal, direct observations. If experts say something that contradicts your experience, listen to them carefully, but ultimately it’s your own data that matters.
“You must not fool yourself — and you are the easiest person to fool.”2
Be quantitative, but not too quantitative.
Read and listen widely — especially to points of view that contradict your current beliefs.
It’s okay to change your mind. “I was wrong and here’s why” is among the most beautiful phrases a personal scientist can utter.
Life is short. “In the long run we’re all dead”. Don’t get stuck in analysis-paralysis.
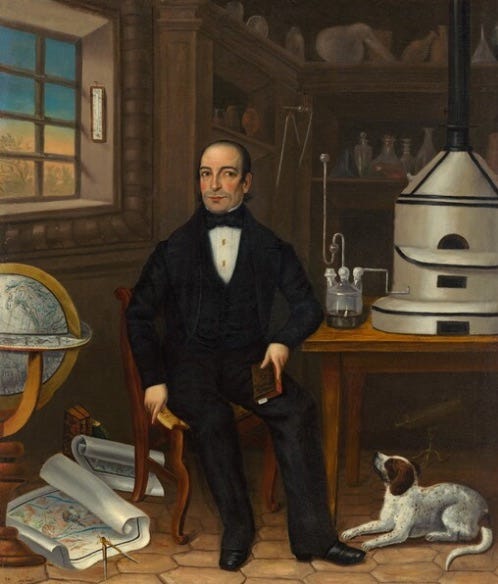