
Personal Science Week - 230914 Statistics
A simple way to confirm if you results are due to chance, plus more links
Personal scientists are open-minded but skeptical, and we tend to be more trustful of quantifiable data. But how can you tell whether you’ve uncovered an interesting result or whether it’s spurious and random?
This week we’ll walk through the simplest technique to quickly eyeball your data to see whether it’s statistically significant.
T-Testing and P-Values
You have a bunch of data and you just want to know quickly if there are any patterns. The simplest way to do this is through a “T-Test”, which you may have learned long ago in school. Here’s a short refresher.
In this example, let’s assume I want to know whether drinking alcohol affects my sleep or not. I’ve measured my sleep for 20 nights. On ten of those nights, I had a glass of wine with dinner. On the remaining ten days, I didn’t.
Specifically, I'll look at whether there is a difference in the amount of sleep I get on nights when I consume alcohol compared to nights when I don't.
Approach:
Data Cleaning: First, I’ll separate the data into two groups based on whether I drank alcohol that night or not.
Descriptive Statistics: Calculate the mean, standard deviation, and other metrics for each group.
Statistical Test: Use an appropriate statistical test to determine if the differences are statistically significant. In this toy example, I’m comparing two equally-sized samples (10 nights each), each of which is considered independently of the other.
If you organize your data like the following chart, the only thing you need to remember is this formula:
=T.TEST(B3:B12,C13:C22,2,2)
Where B3:B12
is the range of cells corresponding to the nights with alcohol and C13:C2
is the range without. The remaining parameters specify that you’re analyzing 2 datasets (“tails”) for which you’ll use a 2-sample equal variance test.
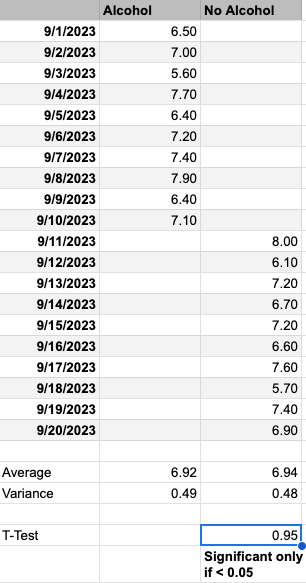
In this (toy) example, the T-Test concludes with a p-value of 0.95. Very roughly, this corresponds to the percentage of time that any differences in the data are due to chance. By convention, scientists typically assume chance for any p-value greater than 0.05 — which means this example’s results can be chalked up to random variation.
There are many nuances in how to properly treat p-values, but for personal science purposes, this is a quick-and-dirty interpretation that tells me I am unlikely to find a pattern.
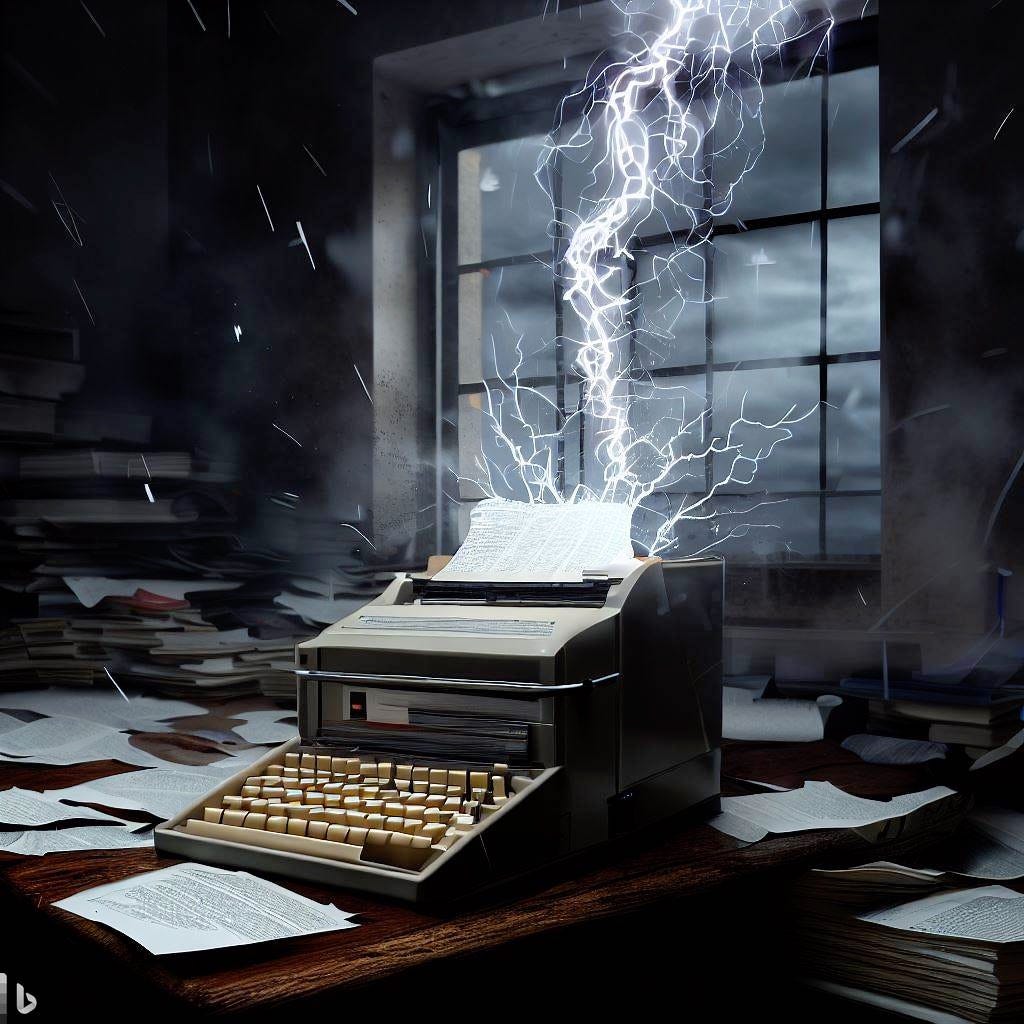
The Fine Print
We didn’t find a pattern in this example, but that’s the point. You’re unlikely to find patterns in most of the data you collect yourself — which is why it can be especially exciting when you do find something.
This T-Test is simple enough and fast enough that I regularly use it to weed out my personal theories that are obviously spurious. Only if I see a p-value under the conventional limit of 0.05 will I bother following up.
Even then, you need to be aware of some important limitations: the conventional 0.05 threshold means that roughly one out of 20 tests will appear to be significant due to pure luck. If you repeat this experiment every day throughout a year, you’ll almost certainly bump into one week where it appears significant purely due to chance.
A more subtle, but potentially serious problem with any time-based experiment like this one is called autocorrelation. The amount of sleep I get on Monday may or may not relate to the amount of alcohol I consumed, but — importantly — it almost certainly does relate to the amount of sleep I had the night before. Unless I correct for that so-called “temporal correlation”, my final results can be very skewed.
There are many statistical techniques to help with autocorrelation, but my advice is to not bother unless you’ve (1) collected a lot of data, and (2) already saw a potential pattern using this simple T-test.
In other words, use the T-Test before continuing with more detailed analysis. If you don’t find significance here, you’ll almost certainly not find it even with a much more complicated approach.
Links Worth Your Time
We enjoyed two excellent podcasts this week:
Econtalk interviewed Anupam Bapu Jena on Random Acts of Medicine. This Harvard economist describes various studies showing how for example cardiac deaths go down on days there are big cardiac conventions. Doctors, especially those with experience and expertise, might be more likely to intervene than those who are less confident; so the more tricky (and likely to fail) edge cases don’t get treated when the specialist is gone because everyone else is too scared to try.
Peter Attia and Andrew Huberman discuss two papers, including one that appears to disprove the idea that metformin helps with longevity, and another that shows how that believing something works can actually generate physiological changes to the brain.
and two more items to consider:
Should I try the carnivore diet? Two biohacker friends recommended I try Paul Saladino’s Heart and Soil freeze-dried beef organ supplements. Grass-fed beef is among the most nutrient dense foods available, and a friend swears by the improvements in blood markers after taking these for a few months.
Before you bother with this year’s flu shot, look at most exhaustive review of the evidence ever conducted. The gold standard in evidence-based surveys, the Cochrane Review, concludes the shots work about as well as … um … that p-value example above. Statistically you might prevent one case if you vaccinate 71 healthy adults, but you might also make them more susceptible to other illnesses. Check this 2019 Dutch study, which compared real flu shots to placebo among a thousand elderly people who were followed for twenty-five years (!). The shots made no difference in life expectancy.
“Our findings suggest people with known risk factors for heart disease and stroke may benefit from having their blood pressure checked while lying flat on their backs,” Giao said. Sept 2023 study from American Heart Association (H/T HN)
As always, if you have any additional information, especially counter-arguments, please let us know! We want to be proven wrong!
About Personal Science
Personal Scientists are skeptical about everything. We follow the 1660 motto of the Royal Society: Nullius in verba, “take nobody’s word for it”. But we’re also open-minded and curious.
Our weekly newsletter is free to everyone. Paid subscribers have access to our “Unpopular Science” series, including our recent one on gender and sex.
For interactive discussions with other Personal Science-minded people (including me), please join the Open Humans Weekly Self-Research Call, every Thursday at 10am Pacific Time. Open to everyone, and very friendly. (See Personal Science Week - 15 Sep 2022).
If you have additional comments or questions, especially about topics you’d like us to cover, please let us know.
Regarding the flu shot: If you're at risk of not surviving the flu because your immune system is weakened, you might also not be gaining much protection from the flu shot, even in years where they got lucky with the target selection. But those same people *do* benefit if the healthy people around them are less likely to infect them. So you'd have to compare death rates at nursing homes where the staff is vaccinated vs not vaccinated. Might be tricky to get an IRB to sign off on that study though 😬